"Deep neural networks work like a Swiss army knife"
Prof. Helmut Bölcskei, Professor of Mathematical Information Science, explains in our interview how mathematics could advance deep learning over the next few years, why artificial intelligence does not scare him and what he particularly appreciates about ETH Zurich.
Prof. Bölcskei, what is your main area of research?
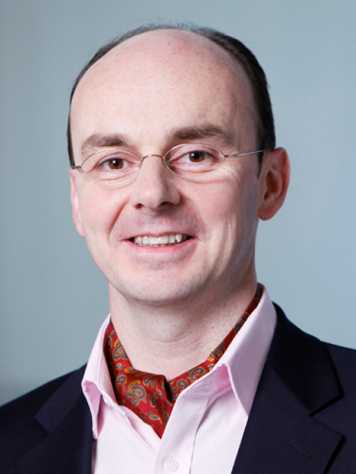
Our main field are the mathematical information sciences, i.e. mathematical theories of information processing systems and their fundamental limits as well as mathematical modelling and the analysis of these models. Currently, we conduct research in the fields of machine learning, mathematical signal processing and statistics.
What brought you to this field of research? Why does it fascinate you?
Since I could not make up my mind when I was in high school and both subjects were very appealing to me, I decided to study a mix of electrical engineering and mathematics. That was exactly the right thing for me, as I had always wanted to work at the interface of these two disciplines. One could say that the same two subjects have fascinated me for 30 years. I wanted to do something that combines exciting applications with beautiful mathematics.
Some time ago your professorship was renamed from "Chair for Communication Theory" to "Chair for Mathematical Information Science". What was the reason?
The renaming reflects the current research focus of my group and could actually have been done much earlier. About ten years ago, my research began to shift to the area of "data science". It became gradually clear that this should be reflected in the name of my research group. Nowadays, it is hardly possible to conduct research in just one field for an entire scientific career because developments have become so fast-paced.
According to the homepage of your research group, you are "currently interested in developing a mathematical theory of ´Deep Learning´". What does that exactly mean?
"Deep Learning" (DL) using deep neural networks has been very successful in applications since the mid-2000s, e.g. in the areas of autonomous driving and computer vision, but comparatively little research has been done on the mathematics behind it. We started our efforts in this area seven years ago. In the meantime, DL has become one of the big topics in applied mathematics. Deep neural networks are mathematical structures that imitate functional structures occurring in nature and thereby build an artificial neural network (ANN). What is new is the way in which mathematical functions are built up. In the past, components would have been assembled like puzzle pieces, today the signals are first rotated and shifted, transformed nonlinearly, then rotated and shifted again and transformed nonlinearly and so on. This process is repeated often, hence the name "Deep Neural Networks".
The main difference to classical methods is the impressive universal learning capability of artificial deep neural networks. Initially, such systems do not contain any information, but they derive information from the examples they are presented with. Through training, an ANN can learn to change the connections within the network so that it can classify unknown data with high accuracy based on the learned "rules". This idea has its origins in biology, it is believed that the human brain could function in this way. Whether or not this is actually the case is not that important for those interested in the underlying mathematics. What is special about deep neural networks is that they can learn a large number of different structures in an optimal manner. Such a statement can even be proven mathematically.
In a lecture you mentioned "AlphaZero", an autodidactic computer programme which can learn complex board games solely on the basis of the rules of the game as well as through intensive playing against itself. It exceeds human performance, for example in the game of "Go". Are you personally afraid of these advances in artificial intelligence?
I'm not really worried about these developments. Almost any technology can be used both for good and bad purposes. Human work will evolve in the future with the help of artificial intelligence. We will be relieved of tedious tasks so that we can concentrate even more on things we really enjoy doing. Our learning will also become more efficient: In 2017, Garry Kasparov coined the motto of "Man with Machine" in his book "Deep Thinking: Where Machine Intelligence Ends and Human Creativity Begins". He said that human chess players could acquire completely new game strategies thanks to insights delivered by the machine and could thereby become stronger and more creative players themselves.
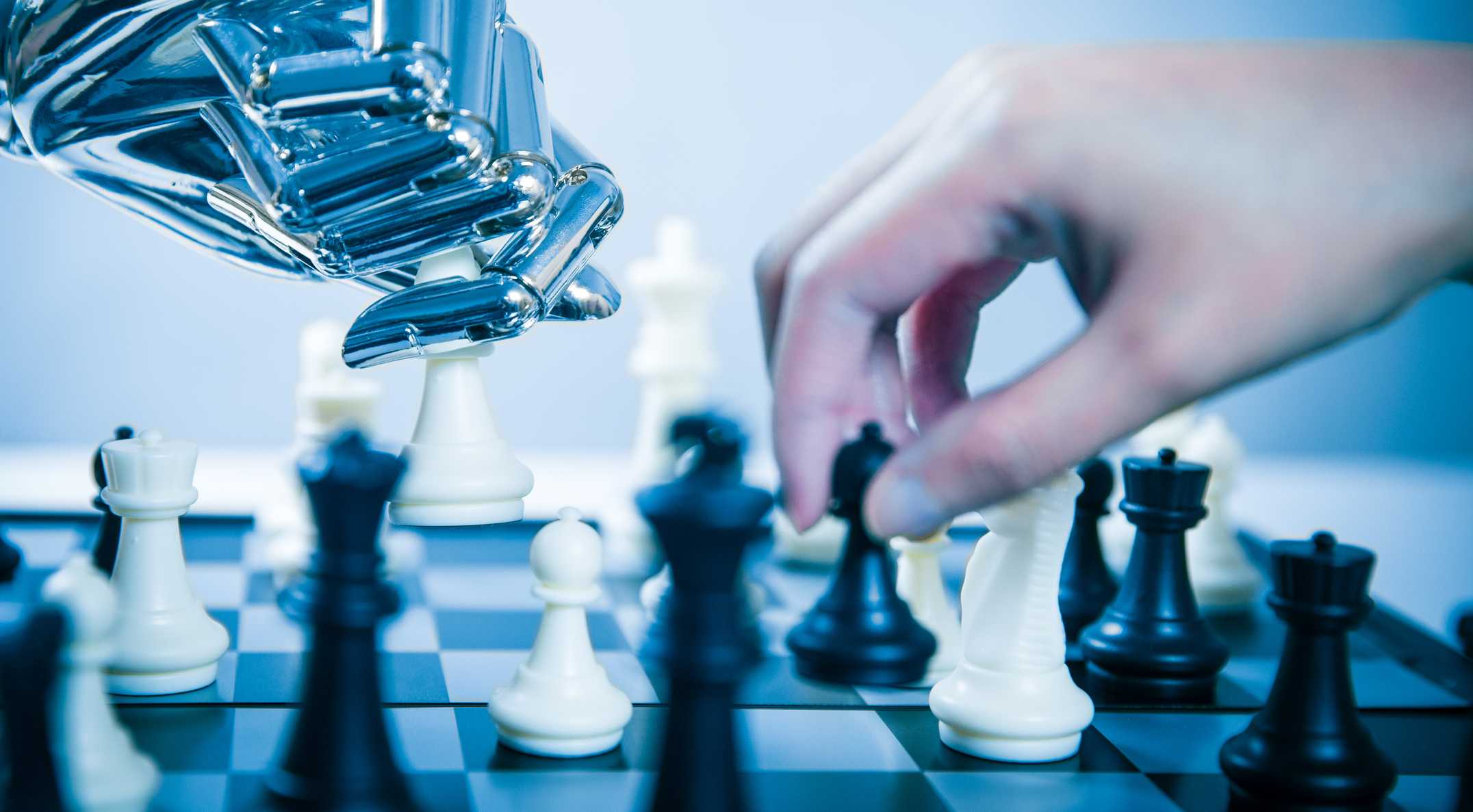
How do you like ETH Zurich as a research institution?
It is excellent! What I particularly like is the freedom we are given in shaping our research group and in the way we conduct research. We can decide ourselves whether and how much we want to work with industry, whether we want to have a large or a small research group, and where we recruit our PhD students from. The density of excellent researchers at ETH Zurich is impressive and the cooperation with the Departments of Computer Science and Mathematics in research and teaching is also very good.
How international is your group? Are you looking for new doctoral students?
I currently have doctoral students and scientific staff from Croatia, Turkey, Ukraine, and Austria as well as a Swiss laboratory technician and a Swiss administrative assistant. Two more doctoral students and a postdoc from France, China, and Uzbekistan will join the group soon. I do not actively look for new students, but wait for good applications from students who share the group's research philosophy. I consider this match to be of utmost importance.
Which lectures will you give in the coming autumn semester?
I will give the undergraduate lecture "Signals and Systems I" for bachelor students in the 3rd semester and a new lecture called "Neural Network Theory" which is aimed at students of mathematics, electrical engineering, computer science, and physics and is also offered in the "Data Science" Master’s programme.
What are currently the biggest challenges in your field of research?
There have been great practical successes in deep learning during this decade. It is now time to develop a corresponding mathematical theory, as the point will be reached where it becomes imperative to theoretically understand why things work so well and in which direction one should proceed. We already know that deep neural networks can theoretically learn everything classical methods can learn and even more, and in all cases optimally so. What is remarkable is that this universal optimality can be attained by a single structure, just like a Swiss army knife. We also know that large networks work better, but do not really understand why. A lot of research will also be needed to fully understand the various learning algorithms. I am looking forward to this interplay between beautiful mathematical theory and exciting practical applications!
Professors at D-ITET
In our interview series, professors at D-ITET give an insight into their research and personal motivation to go into academia.
Links
Chair for Mathematical Information Science
external page«Fundamental limits of deep neural network learning» Talk held at the Erwin Schrödinger International Institute for Mathematics and Physics, (ESI), Vienna, August 2019